Active projects and challenges as of 20.12.2024 14:40.
Hide full text Print Download CSV Data Package
Living Herbarium
(2+6) Connecting the Herbarium to Wikidata around playful experiences and storytelling
We have merged Challenge 2 - Designing Wikidata and Challenge 6 - historicalMAP, and are developing concepts to involve more people, stimulate public awareness and engagement, and create educational materials about the natural world.
Cover photo: Michele Jurietti
1. Figma Concept 2. Leaflet/Wikidata Demo
https://twitter.com/OpendataCH/status/1588625791876988929
Design Notes
Our initial brainstorm is clustered around three topics: Location/Mapping, Specie(s) and History/Change. We collected ideas of experiences and working steps we wanted to achieve at the hackathon.
Using a Miro board we further elaborated our designs, used a collage to organise our ideas, and started putting together a workflow based on which user interaction can be developed.
Figma Concept App
We are now elaborating the interaction design using Figma, which you can preview here:
Data preparation
We are a small team with about a 50/50 split of technical and design background. None of us are domain experts, but we received detailed guidance and had some very helpful and inspiring meetings with our expert and data provider Alessia Guggisberg who works at the ETH and maintains Vascular plants datasets. We are also in contact with another team working on this data:
https://hack.glam.opendata.ch/project/123
They downloaded and kindly helped us to get a first look of the data, which is too large to work with in a spreadsheet program. In parallel, we looked into the way WikiSpecies data is structured on Wikidata, and read up on the import mechanisms for making contributions. There has been prior work in bridging GBIF and Wikidata, which we wished to leverage.
💡 Read more in Does Biodiversity Informatics 💘 Wikidata?
Using OpenRefine and Frictionless Data Creator we have prepared a schema file comapatible with open data tools. This is a Data Package based on a new extract from GBIF. It is filtered to three species with good coverage (around 15'000 observations) in Switzerland, as recommended to us by Alessia.
https://raw.githubusercontent.com/we-art-o-nauts/living-herbarium/main/datapackage.json
Leaflet/Wikidata live demo
Based on this we were able to create a simple web app, which displays a Leaflet map with locations pulled in from a Data Package, and photos of species from Wikidata APIs. The data can be easily updated / filters expanded, and based on this demo app further work is possible.
Screenshots:
Our work continues on GitHub - click the Source link for details.
Next steps
- Meet with our expert next week to discuss results.
- Get support for further design & development work.
- Discuss our results with the Wikidata community
- Connect with citizen science (iNaturalist) communities
- Explore outdoor sensing (TTN) projects.
- Get inspired by cool museum exhibits (Lenzburg)
- Keep hacking on biodiversity data!
Living Herbarium
A Data Package that is part of a #GLAMhack 2022 project to explore an extract from the Swiss National Databank of Vascular Plants. This dataset was recommended and queries provided by Dr. Alessia Guggisberg at the Institute of Integrative Biology (IBZ), ETH Zurich.
For more details visit our Project Page
Notes
We removed all empty / repetitive columns from the dataset. This is therefore not a 1:1 complete export, and you should visit the corresponding GBIF query.
In order to respect the currently nationally agreed ethical framework while simultaneously sharing scientifically utilizable data for large scale studies, Swiss biodiversity data is generally published generalized to 5km grid squares. Altitude information corresponds to raw data.
Attribution
Data citation:
GBIF.org (05 November 2022) GBIF Occurrence Download https://doi.org/10.15468/dl.v8r2q5
Datasets:
- United Herbaria of the University and ETH Zurich
- Swiss National Databank of Vascular Plants
Usage Rights:
CC BY 4.0 http://creativecommons.org/licenses/by/4.0/legalcode
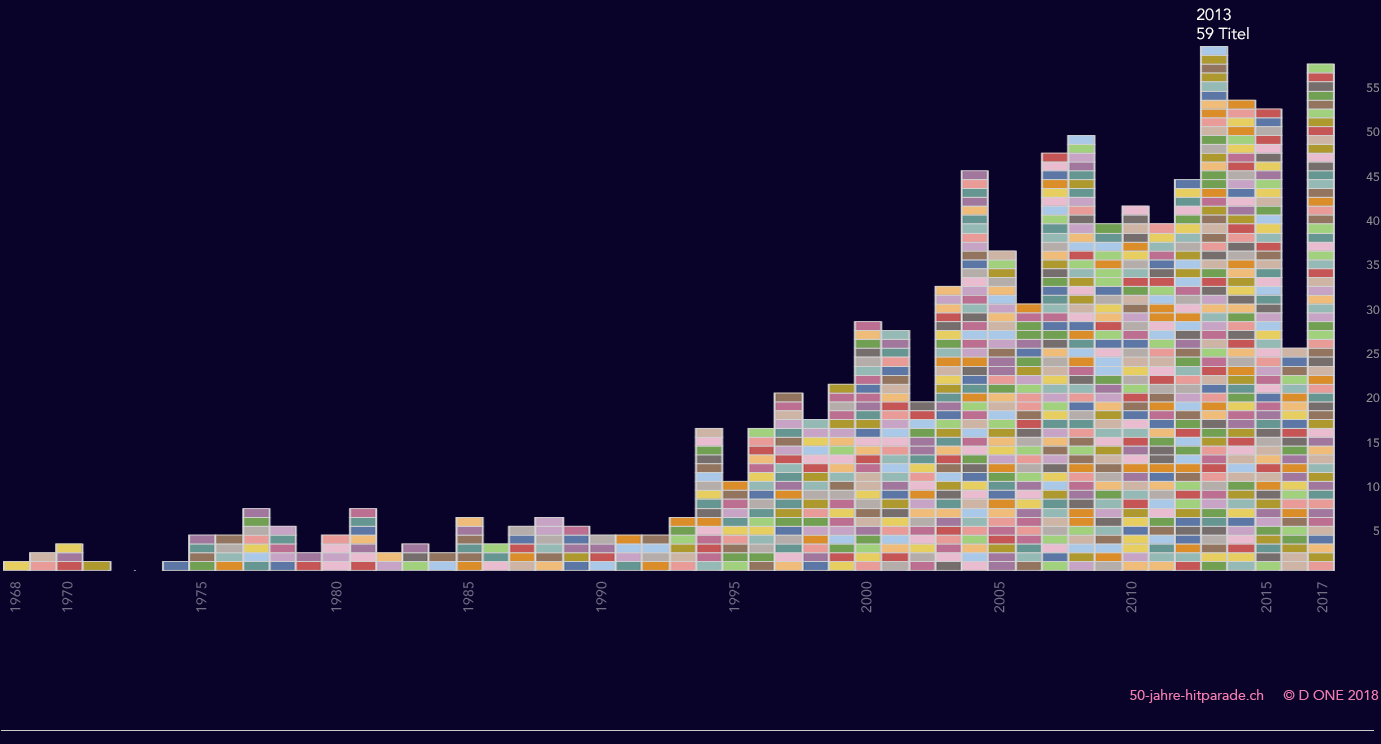
Audio Analysis Challenge
(07) Retrieve as much information as possible from an audio collection, through various Machine Learning/Natural Language Processing methods
https://youtu.be/6_eYklTp37o?t=1006
https://twitter.com/OpendataCH/status/1588814248859545600
Challenge
Retrieve as much information as possible from an audio collection, through various Machine Learning/Natural Language Processing methods:
- speech-to-text
- speech emotion recognition / sentiment analysis (from the transcription text or directly on audio, if doable): classify and tag speech/speakers’ sentiment based on their polarity (positive, negative, or neutral) or beyond (different emotions)
- eventually data visualizations based on the results (e.g., https://50-jahre-hitparade.ch/analysis/ - from where the chart above comes from)
Dataset
Collection “Radio pleine lune”: Radio Pleine Lune, was a feminist radio program in the Geneva region that started with pirate broadcasts in 1979. The collection has been deposited in the Archives contestataires in Geneva, which collects, preserves, and valorizes documents from social movements of the second half of the 20th century. The program existed from 1980 to 1999. It is of particular importance for the Archives contestataires insofar as it gives an account of the various media forms used by protest movements in the second half of the 20th century. The materials represent broadcasts, thus direct recordings in the studio, as well as some rush documents, essentially interviews.
Information about the collection:
http://inventaires.archivescontestataires.ch/index.php/fonds-radio-pleine-lune https://memobase.ch/fr/recordSet/acc-001
Metadata:
https://api.memobase.ch/record/advancedSearch?q=isPartOf:mbrs:acc-001 Metadata are in French. Most relevant fields are the title, the abstract and the keywords (hasSubject).
Data: 443 audio recordings.
Possible issues:
- not enough training data
- chaotic corpus (multiple voices, live speaking)
Needs: developers with experience with audio analysis algorithms; eventually, web designers.
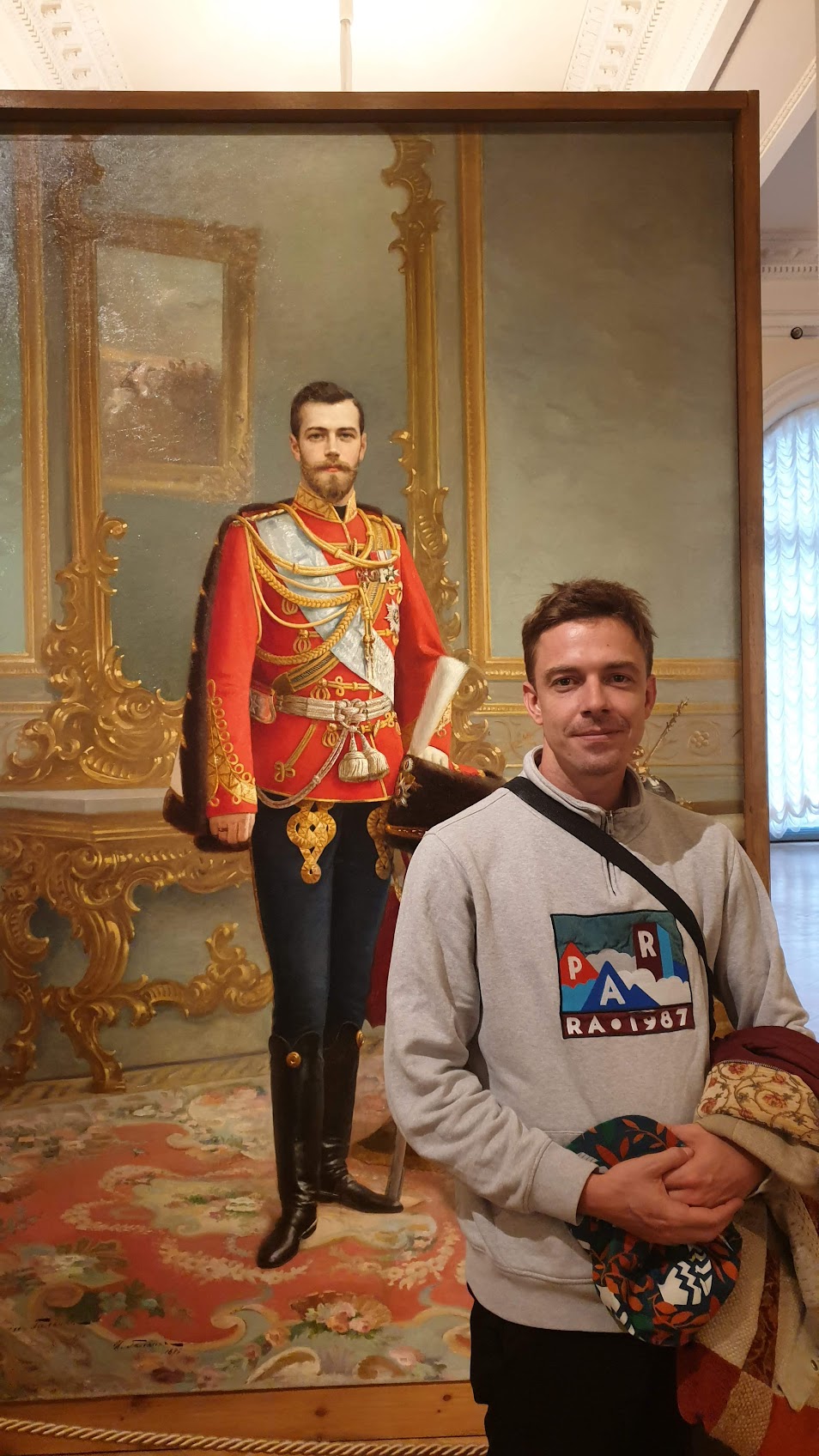
Find my classical doppelgänger
(15) A website where a selfie can be uploaded and the closest classical portrait from the kunstmuseum basels archive will show up
PaintPoserFrontend
This project was generated with Angular CLI version 11.0.5.
Development server
Run ng serve
for a dev server. Navigate to http://localhost:4200/
. The app will automatically reload if you change any of the source files.
Code scaffolding
Run ng generate component component-name
to generate a new component. You can also use ng generate directive|pipe|service|class|guard|interface|enum|module
.
Build
Run ng build
to build the project. The build artifacts will be stored in the dist/
directory. Use the --prod
flag for a production build.
Running unit tests
Run ng test
to execute the unit tests via Karma.
Running end-to-end tests
Run ng e2e
to execute the end-to-end tests via Protractor.
Further help
To get more help on the Angular CLI use ng help
or go check out the Angular CLI Overview and Command Reference page.
Fonoteca Jukebox
(01) An innovative way for visitors of the Swiss National Sound Archive to listen to the 500+ recordings of the Gramophone Collection
https://youtu.be/6_eYklTp37o?t=82
https://twitter.com/OpendataCH/status/1588865199968514050
Resources
Gramophone collection: https://www.fonoteca.ch/cgi-bin/oecgi4.exe/inet_fnbasehrextract?LNG_ID=ENU
Dataset:
https://opendata.swiss/de/dataset/gramophone-collection-swiss-national-sound-archives
This could be used to replace the current listening stations at the Fonoteca Nazionale :
Contact: giuliano.castellani at nb.admin.ch
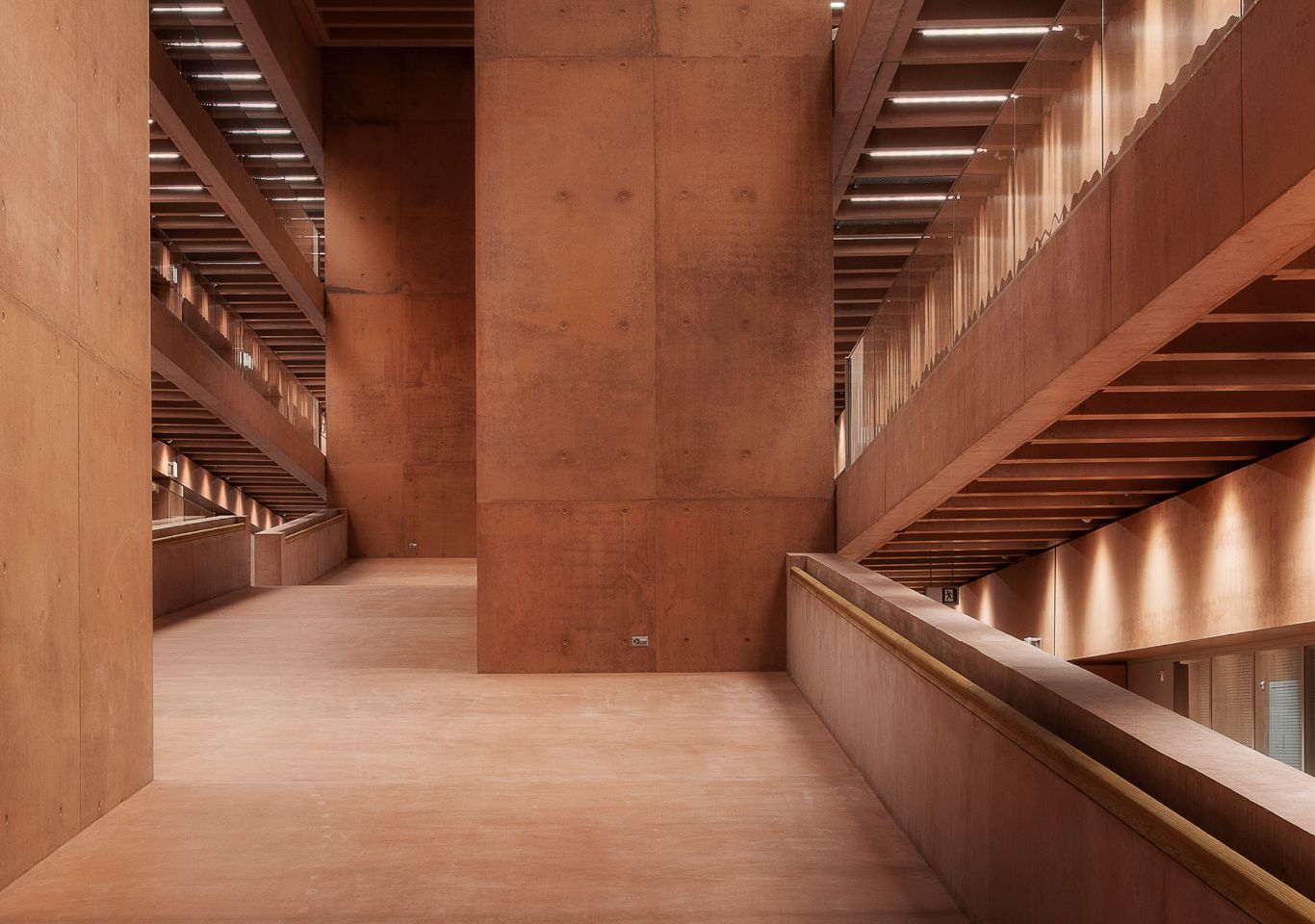
#GLAMhack22 Video
Creative content to encapsulate the essence of the hackathon
RSI Radiotelevisione svizzera - 05.11.2022: GlamHack, come rendere più aperti i dati della cultura
https://www.youtube.com/watch?v=6_eYklTp37o&feature=youtu.be
https://twitter.com/OpendataCH/status/1588471593264885761
Instructions
Let's collect creative content of the event under CC licenses for a shared memory of the experience. Think of the most epic and impactful image, sound or text..then get your artist hat on and make it!
1. Upload content and license your work
We recommend using open platforms toeasily upload clips and stills, from which we will create a "best of" video that will recognise your contributions. Here is a handy list from Creative Commons:
🎯 https://creativecommons.org/share-your-work/places-to-share/
🔜 https://creativecommons.org/platform/toolkit/
2. Let us know
Our team is compiling your videos and submissions. To make sure we are aware of your contributions, please use the "Comment" button to add a link to your collection (or one or two individual items) on this platform. If would like to have your content featured, please ping us on Slack!
🍩 If you are sharing on social media as well, remember the hashtag #GLAMhack22
3. Selected posts
Stay tuned for a collection of outputs from the GLAMhack 2022 event - including a broadcast in Italian from local media! You can already look through Wikimedia Commons, Mastodon, Twitter for live coverage.
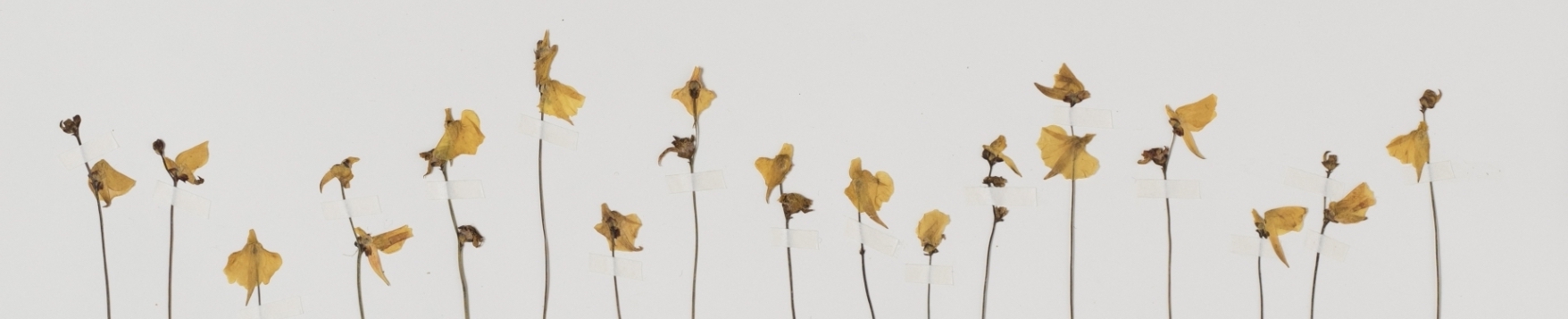
Label Recognition for Herbarium
(14) Search batches of herbarium images for text entries related to the collector, collection or field trip
https://youtu.be/6_eYklTp37o?t=1935
https://twitter.com/OpendataCH/status/1588871226516451328
Goal
Set up a machine-learning (ML) pipeline that search for a given image pattern among a set of digitised herbarium vouchers.
Dataset
United Herbaria of the University and ETH Zurich
Team
- Marina
- Rae
- Ivan
- Lionel
- Ralph
Problem
Imaging herbaria is pretty time- and cost-efficient, for herbarium vouchers are easy to handle, do not vary much in size, and can be handled as 2D-objects. Recording metadata from the labels is, however, much more time-consuming, because writing is often difficult to decipher, relevant information is neither systematically ordered, nor complete, leading to putative errors and misinterpretations. To improve current digitisation workflows, improvements therefore need to focus on optimising metadata recording.
Benefit
Developing the proposed pipeline would (i) save herbarium staff much time when recording label information and simultaneously prevent them from doing putative errors (e.g. attributing wrong collector or place names), (ii) allow to merge duplicates or re-assemble special collections virtually, and (iii) would facilitate transfer of metadata records between institutions, which share the same web portal or aggregator. Obviously, this pipeline could be useful to many natural history collections.
Case study
The vascular plant collection of the United Herbaria Z+ZT of the University (Z) and ETH Zurich (ZT) encompasses about 2.5 million objects, of which ca. 15% for a total of approximately 350,000 specimens have already been imaged and are publicly available on GBIF (see this short movie for a brief presentation of the institution). Metadata recording is, however, still very much incomplete.
Method
- Define search input, either by cropping portion of a label (e.g. header from a specific field trip, collector name or stamp), or by typing the given words in a dialog box
- Detect labels on voucher images and extract them
- Extract text from the labels using OCR and create searchable text indices
- Search for this pattern among all available images
- Retrieve batches of specimens that entail the given text as a list of barcodes
Examples
Here are some images of vouchers, where what could typically be searched for (see red squares) is highlighted.
a) Search for "Expedition in Angola"
b) Search for "Herbier de la Soie"
c) Search for "Herbier des Chanoines"
d) Search for "Walo Koch"
e) Search for "Herbarium Chanoine Mce Besse"
Problem selected for the hackathon
The most useful thing for the curators who enter metadata for the scanned herbarium images would be to know which vouchers were created by the same botanist.
Vouchers can have multiple different labels on them. The oldest and most important one (for us) was added by the botanist who sampled the plant. This label is usually the biggest one, and at the bottom of the page. We saw that this label is often typed or stamped (but sometimes handwritten). Sometimes it includes the word 'leg.', which is short for legit, Latin for 'sampled by'.
After that, more labels can be added whenever another botanist reviews the voucher. This might include identifying the species, and that could happen multiple times. These labels are usually higher up on the page, and often handwritten. They might include the word 'det.', short for 'determined by'.
We decided to focus on samples that were sampled by the botanist Walo Koch. He also determined a lot of samples, so it's important to distinguish between vouchers with 'leg. Walo Koch' and 'det. Walo Koch'.
Data Wrangling documentation
How did we clean and select the data ?
Selected tool
We decided to use eScriptorium to do OCR on the images of the vouchers. It's open source and we could host our own instance of it, which meant we could make as many requests as we wanted. We found out that there is a Python library to connect to an eScriptorium server, so hopefully we could automate processing images.
Setting up escriptorium on DigitalOcean
eScriptorium lets you manually transcribe a selection of images, and then builds a machine-learning model that it can use to automatically transcribe more of the same kind of image. You can also import a pre-existing model. We found a list of existing datasets for OCR and handwriting transcription at HTR-United, and Open Access models at Zenodo.
From the Zenodo list, we picked this model as probably a good fit with our data: HTR-United - Manu McFrench V1 (Manuscripts of Modern and Contemporaneous French). We could refine the model further in eScriptorium by training it on our images, but this gave us a good start.
Video Demo
Presentation
Google Drive folder
Tools we tried but where we were not successful
Transkribus
https://readcoop.eu/transkribus/. Not open source but very powerful. Not easy to use via an api.
Open Image Search
The pipeline imageSearch developed by the ETH Library. The code is freely available on GitHub and comes with a MIT License.
We were not able to run it, because the repository is missing some files that are necessary to build the Docker containers.
Github
See also our work on Github, kindly shared by Ivan.
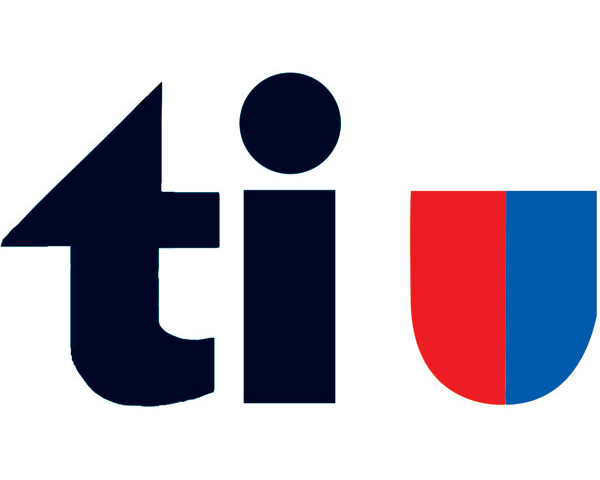
Mappa Letteraria
(13) Paving the way for more open data in Tessin
Better information for tourists so they can better know what they are seeing as they are moving from site to site. The result is an initial exchange of information, we better understood that from the database of the Canton significant data could be obtained, uploaded to Wikidata (similar to Noon at the Museum) and used for innovative applications.
https://hack.glam.opendata.ch/project/172
https://youtu.be/6_eYklTp37o?t=1730
https://twitter.com/OpendataCH/status/1588877340851195905
📦 File: Presentation UAPCD Hergys Helmesi_compressed.pdf
#glamhack2022 #openglam #ar #mobile
Mont
(05) Mont is an AR webapp that lets you discover the hills and mountain tops around you.
Swiss geodata (cartographic, topographic, toponymic), once Switzerland's most protected data (for reasons of military defence), have become freely available in the past years. At the same time webtech has greatly evolved. Today mobile webpages can access many of the hardware components built into modern smartphones, such as camera, gyroscope, and compass modules. This allows for coding Augmented Reality apps that will run in any web browser and on any smartphone.
MONT is a Javascript-driven web app that lets you discover the hills and mountain tops around you. Point to any hilltop within sight, tap on the red button and get the hill or mountain name, its distance and height above sea level.
MONT is a Augmented Reality web app coded in "vanilla" JS. It is a proof of concept in order to demonstrate that AR applications for mobile phones can be done just by using open technologies such as HTML 5 (CSS 3, Javascript ES 6).
Processing
After geolocating the app processes a digital height model of Switzerland and interpolates the current height above sea level. It then triangulates the mountain peak or hilltop in question (horizontally and vertically) and searches the mountain database in order to determine the precise peak the user is pointing to.Tech
MONT is coded in Javascript only ("vanilla" JS, hence no external dependencies, no frameworks, no libraries, no third-party services of any kind). MONT is a so-called flat-file webapp, which means that the required data (digital height model, toponymy and georeferences) are stored in JS arrays (instead of a database) that are cached by the web browser, so that the app, once loaded, will also work in offline mode.Data
- Digital height model of Switzerland (200m grid)
- Swiss geographical Names (georeferenced)

Compared to programming native applications for specific platforms, using web technologies has two major advantages.
- Cross-platform compatibility: Functionality and design will work across all platforms, no matter what operating system (or device).
- No app installation: Developers do not need any app distribution service or app store. As soon as the web app is uploaded to a web server, it is accessible to any user. A link or a qr code is everything needed.

Yet, there are a couple of downsides to the MONT project.
- Compass heading (1): The HTML geolocation API according to the W3 web standard includes a
heading
property indicating the user's (i.e. the smartphone's) absolute orientation in degrees. Yet, despite of being part of the web standard this property has never been implemented. Theheading
property retrieved by thegetCurrentPosition()
method returnsnull
. - Compass heading (2): iOS and Android handle web browser access to orientation data differently. While iOS delivers absolute compass data (by means of the non-standard
webkitcompassheading
property), Android only delivers rotation data that set 0 (i.e. North) to the direction the smartphone was heading towards when the page was loaded. - Sensor inaccuracy: Motion and compass sensors (magnetic compass module, motion sensors/gyroscope) built into modern smartphones are quite inaccurate. While there can be several mountain peaks within one single compass degree (especially given the MONT reach set to 20 kilometers), even a slight inaccuracy can lead to false results. This may change with future improvements to sensor technology.
Team:
- Prof. Thomas Weibel, University of Applied Sciences of the Grisons (FHGR), Berne University of the Arts (HKB)
- Dirshti Gopwani, University of Applied Sciences and Arts of Southern Switzerland (SUPSI)
Credits
Swiss Federal Office of Topography (swisstopo)Social media
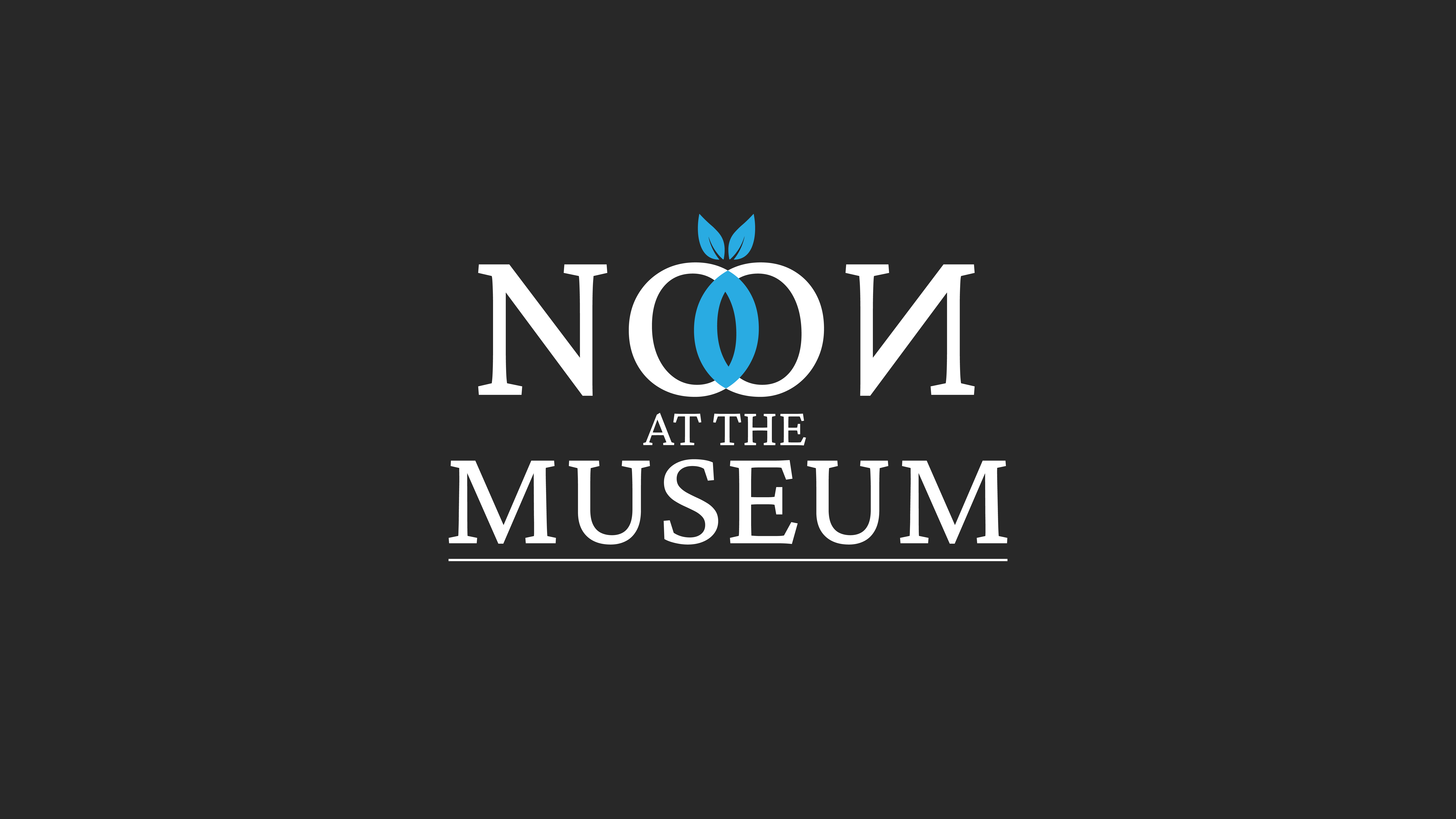
#GLAMhack2022 (hashtag for OSM)
Noon at the Museum
(12) Despite excellent exhibitions about future foods and ecological diets: museum restaurants often disappoint. Let's change this together!
https://youtu.be/6_eYklTp37o?t=1361
https://twitter.com/OpendataCH/status/1588839232273276928
Motivation
Curatorial practices and mediation programs are increasingly shaped by core concepts of sustainability, equity, social justice and diversity, and – according to the new 2022 ICOM museum definition – “accessible and inclusive, museums foster diversity and sustainability”. Still, just one step outside of the exhibition rooms, in museum shops and museum cafeterias, remnants of past worlds such as exclusionary dietary options or gemstones of questionable origin sold in museum shops are presented to the visitors, forming a sharp contrast to the intellectual menu offered by these institutions.
The challenge “noon at the museum” aims at addressing museum sustainability and institutional responsibility. It focuses on museum restaurants and their surroundings to answer the simple question for vegetarians and vegans “Can I eat here?”. It relies on open and crowd-sourced data to document the current existing situation in Canton Ticino, to improve the situation and to design a tool and a process for the future.
© OpenStreetMap contributors, visualization based on "Is OSM up-to-date?" by Francesco Frassinelli, adjusted for the GLAMhack2022
Hackathon challenge, prototype and how-to
As a prototype of the concept, our scope falls on museums in Ticino. We provided answers to the following questions:
- How many museums have a restaurant?
- Do they offer at least one vegan/vegetarian option?
- Can we find vegan/vegetarian options nearby (within a 50m radius around the museum)?
- Can we identify a system which will allow us to have access to this information in the future?
Within this challenge we documented our research and development process and we captured workflows and discussions as they unfolded.
Process documentation
Summary of the prototype (Ticino Canton)
- Identification. We identified the information about restaurants and museums which can be included in OpenStreetMap. OpenStreetMap doesn’t allow to enter information on the menu-level, however, it allows to embed an URL to the menu and provides multiple fields that give a summary over the whole. There is a “diet type” field available in OpenStreetMap that allows for various tags like “vegan”/”vegetarian”/”gluten-free”/… These tags can further have four different values: Yes, No, Only, (limited). There is no field or tag indicating whether explicit information on the ingredients is included in the menu.
- Data extraction from OpenStreetMap. Using OpenStreetMap it is possible to query the map data for restaurants and cafeterias with vegetarian and vegan lunch options located within 50m from museums. At first there were no results for 50 m around museums in Canton Ticino; at the end of the GLAHack the results were 6 restaurants with vegetarian options (4 also vegan) over a total of 21 restaurants (please refer below; search-query); within 200 m radius we found 14 vegetarian restaurants (9 also vegan) at the end of our work over a total of 134 restaurants (search-query).
- Data related to museum restaurants and cafeteria. We searched museums.ch (which has the option to search for museum with a restaurant or a cafeteria in a specific canton) which currently lists 6 museums meeting the criteria; we collected information from people living in the area who know the museums. We found 12 museums with a restaurant or cafeteria, as well as 2 museums which explicitly refer to picnic sites in their website.
- Checking diet options: We checked the menus available online and we called 5 restaurants to check for vegetarian or vegan options. The best results with the calls were collected from a person with experience in calling restaurant to organise events. 6 restaurants have vegetarian and vegan options explicitly mentioned in their menu or in their restaurant description. By calling, restaurants provided availability in providing vegetarian and vegan options, but they were not included in OpenStreetMap; 1 restaurant reported availability in providing vegetarian options only.
- Data related to museums on Wikidata. On Wikidata there are 118 museums of Canton Ticino uploaded in 2018. We checked the data and we improved it (fixing broken links, completing names in English, fixing redundancy in administration units); we added information about restaurants, shops and picnic sites in the property P912 and we suggested to the Wikidata communities to include this property in the project Museum (please refer to the discussion page)
- Data related to museums on OpenStreetMap. On OpenStreetMap we updated information related to the restaurants and cafeteria; we used and tested the how-to document produced (see below). All changes done during the team during the hackathon were tagged with the hashtag #GLAMhack2022 and can be found on OSMCha.
Reproducibility and How-to
We wrote, illustrated and tested a how-to document for potential future contributors. The document guides them step-by-step through the workflow of tagging the available diet options of restaurants in the vicinity of a museum.
Results
The work implemented allowed to create a simple tool and process, which relies on open and crowd-sourced data and which provides access to information related to vegetarian and vegan options in restaurants in and around museums. This process can be extended to other features of restaurants and to features of museum shops.
The focus on Canton Ticino allowed to improve open data related to cultural institutions and to enrich them with reference to restaurants and shops.
Discussion
The work highlighted how restaurants and shops are indeed not considered an integrated part of museum strategies and design. They are often not presented in their websites and there is a very limited attention to a sustainable, accessible and inclusive approach.
Furthermore working on the challenge arose the following questions:
- Data quality
- How to define ”vegan enough”? How is this reflected in the current tagging model of OpenStreetMap (see their wiki)
- What are the limits of tags/labels? What are good practices in terms of approval, updating-frequency or degree of detail? How to reflect this in the context of greenwashing?
- How to reflect more complex sustainability-indices (regional, seasonal, organic, …)?
- Finding museum restaurants
- How accurate are existing lists and maps?
- How to query-search for museum-restaurants?
- Reproducibility
- How to document the process?
- How to link and reconcile with pre-existing documentation and advice?
- Usability
- What may possible user journeys look like?
Credits and contact information
Working group during hackathon
- Levyn Bürki (proposal of the challenge, structure of the challenge, documenting the process, testing of the how-to)
- Paul Brunner (OpenStreetMap data extraction, check OpenStreetMap policies and data)
- Iolanda Pensa (enrich data related to museums in Canton Ticino on Wikidata, adding property P912 to museum with restaurants; adding some data on OpenStreetMap; research on museum restaurants and menus; report)
- Erzsébet Tóth-Czifra (text with the structure of the challenge, testing of the how-to)
- Camila Méndez (working on the project logo and visual, printed prototype)
- Matteo Subet (research on museum restaurants and menus, testing of the how-to)
- Kristina Beljan (research on museum restaurants and menus)
- Anna Saini (research on museum restaurants and menus)
- Valerio Bozzolan (Wikidata queries)
- Stefano Dal Bo (enrich data related to museums in Canton Ticino and Wikidata queries)
- Luca Landucci (enrich data related to museums in Canton Ticino)
- OpenStreetMap contributors (map data)
Data released under CC 0; the rest of content is released under the CC BY-SA 4.0 licence. Attribution: GLAMHack - Noon at the Museum 12, SUPSI, Mendrisio 2022.

Semi-automatic tagging of images on Wikimedia Commons
(17) Help us test the enhanced version of the ISA Tool, a crowdsourcing tool for tagging images on Commons
https://youtu.be/6_eYklTp37o?t=2629
Description of the tool: https://commons.wikimedia.org/wiki/Commons:ISA_Tool
Testing / discussions to focus on:
- Design, ease of use of the tool
- What is considered "good" tagging? - Best practices in adding "depicts" statements on Commons; instructions; challenging cases
- Usage scenarios / use cases for the data generated
- Assessment of the tool from the point of view of heritage institutions - use by own staff members, carrying out crowdsourcing campaigns
ISA Tool - User Tests in the Context of GLAMhack 2022
Documents / Links:
Usability Tests could focus on:
- Look, feel, and usability of the tagging tool with the machine vision / metadata-to-concept enhancements in place.
- Usefulness of the tool from the point of view of the Wikimedia Commons community (alignment with community norms, helpfulness in getting the ‘work’ done, etc.)
- Usefulness of the structured data that is being generated thanks to the tool from the perspective of GLAM institutions (e.g. in the context of content donations).
The involvement of GLAM staff in the project would be most helpful with regard to 1 and 3.
- The level of involvement will depend on their availability, readiness, personal background, prior experience, organizational context, etc. – Forms of involvement may include:
- “Play” around with the ISA Tool, make a few edits, and give us feedback from the point of view of an occasional user (with or without prior experience as a contributor to Wikimedia Commons).
- Make a substantial contribution to the tagging of a collection / campaign (several hours of work with the tool, possibly exchanges with the community), and give us feedback from the point of view of an advanced user of the tool. Assess to what extent they could imagine that someone at their institution would use the tool for the tagging of their own image collections.
- Assess the usefulness of the structured data generated with the help of the tool. If possible: Compare it to other tools / other approaches which they are familiar with.
- Help us identify / provide data that could be used for benchmarking the quality of the tagging on Wikimedia Commons with the help of the ISA Tool against other algorithm-supported tagging tools (automatic or semi-automatic). – This would require that the given institution already has used such approaches on their own image collections that are available on Wikimedia Commons.
- Evaluate the desirability of using the ISA Tool as the basis for their own crowdsourcing campaigns. – This would require that the given institution already has carried out or at least given some thought to carrying out their own crowdsourcing campaigns.
User stories / usage scenarios
Challenges

historicalMAP
Build interactive maps in HTML format to assess changes in species distribution through time
Projects
https://hack.glam.opendata.ch/project/177
This was presented as Challenge 6 at GLAMhack 2022.
Challenge
Develop a plugin to compare the distribution of (mainly historical) observations from natural history collections with approximate (mainly contemporary) data from Swiss biodiversity portals.
Problem
Swiss biodiversity portals like InfoSpecies publish most of their georeferenced data as 5km square grids in GBIF (see for example here). In order to compare these contemporary data with georeferenced historical data from natural history collections, the latter must be ‘normalised’ to the square grid system used by Swiss biodiversity portals.
Benefit
Comparisons between historical and contemporary observational data allow the assessment of changes in species distributions over time. In the context of the current biodiversity crisis, facilitating such comparisons would tremendously facilitate the identification of threats to the Swiss indigenous flora, fauna and fungi and, by implication, the actions needed to preserve them.
Case study
The vascular plant collections of the United Herbaria Z+ZT of the University (Z) and ETH Zurich (ZT) encompass about 2.5 millions herbarium specimens (see this short movie for a brief presentation of the institution). Between 2019 and 2021, all (ca. 90,000) vouchers from the canton of Valais have been imaged and publicly released in GBIF. More than half (ca. 55’000) of the specimens were transcribed and georeferenced for a total of nearly 1,200 taxa occurring in Valais. These historical data could now be compared with ongoing vegetation surveys from the FloraVS project, which are being published on GBIF via InfoFlora.
Basic approach
- Specify taxon in search field
- Extract historical data of the given taxon from the United Herbaria Z+ZT
- Extract contemporary data of the given taxon from InfoFlora
- Normalise all data to 5km square grids
- Create distribution map for the time period before and after 1980
Jump start
I suggest to use the open-source JavaScript library called Leaflet to solve this challenge. I tried it using R (instead of Java, which I do not know how to program) and herewith briefly summarise what I have already been able to achieve with a few screenshots.
Trial dataset
Distributional data of Adonis aestivalis L. from canton Valais
Link to contemporary data from InfoFlora using following filters:
- Scientific name = Adonis aestivalis L.
- Basis of record = Human observation (to avoid including data from herbaria or literature)
- Administrative areas = Valais - CHE.23_1
- Dataset = Swiss National Databank of Vascular Plants
Link to (mainly) historical data from the United Herbaria Zurich Z+ZT using following filters:
- Scientific name = Adonis aestivalis L.
- Administrative areas = Valais - CHE.23_1
- Dataset = United Herbaria of the University and ETH Zurich
R code
#############################################################################
## Script to assess changes in species distribution using interactive maps ##
#############################################################################
## Code by Alessia Guggisberg
## Created: 18.10.2022
## Last edited: 21.10.2022 by Alessia Guggisberg
## helpful links
## https://rpubs.com/huanfaChen/grid_from_polygon
## https://rstudio.github.io/leaflet/
## https://cran.r-project.org/web/packages/leaflet/leaflet.pdf
## https://leaflet-extras.github.io/leaflet-providers/preview/
## https://leanpub.com/leaflet-tips-and-tricks/read
## https://api3.geo.admin.ch/services/sdiservices.html
## https://leaflet-tilelayer-swiss.karavia.ch/layers.html#
## https://mikejohnson51.github.io/leaflet-intro/hospitals.html # to customised legends
## Initialize libraries and set working directory ######################################################
library(rgdal)
library(leaflet) # to create interactive maps
library(leaflegend)
library(htmlwidgets) # to save interactive maps as HTML
setwd("/Users/guggisberg/Documents/Alessia/Professional/Herbarium/Projekte/GLAMhack2022/Rscript/")
## Import historical Z+ZT and contemporary Infoflora data from GBIF #####################################
INFOFLORA <-read.table("Adonis_aestivalis_0101032-220831081235567/occurrence.txt", header=T, sep = "\t", quote = "\"'")
## Build raster #########################################################################################
grid_size <- 0.055
r <- raster(xmn=5.956601, ymn=45.81914, xmx=10.49347, ymx=47.80988, res=grid_size)
r[] <- 0
crs(r) <- CRS("+init=epsg:4326") # add CRS to raster
rZZT <- rasterize(ZZT[,c("decimalLongitude","decimalLatitude")], r, fun = "count")
rINFOFLORA <- rasterize(INFOFLORA[,c("decimalLongitude","decimalLatitude")], r, fun = "count")
# Retain only grids that contain a count and 'normalise' to centroids of 5km-by-5km grids ###############
rZZT[rZZT < 1] <- NA
rINFOFLORA[rINFOFLORA < 1] <- NA
cellsZZT <- which(is.na(rZZT@data@values)==F)
cellsINFOFLORA <- which(is.na(rINFOFLORA@data@values)==F)
centroidsZZT <- data.frame(xyFromCell(rZZT, cellsZZT))
centroidsINFOFLORA <- data.frame(xyFromCell(rINFOFLORA, cellsINFOFLORA))
# URLs to various Swiss maps ############################################################################
url1 <- 'https://wmts.geo.admin.ch/1.0.0/ch.swisstopo.pixelkarte-farbe/default/current/3857/{z}/{x}/{y}.jpeg' # swisstopo colour
url2 <- 'https://wmts.geo.admin.ch/1.0.0/ch.swisstopo.pixelkarte-grau/default/current/3857/{z}/{x}/{y}.jpeg' # swisstopo greyscale#
url3 <- 'https://wmts.geo.admin.ch/1.0.0/ch.swisstopo.swissimage/default/current/3857/{z}/{x}/{y}.jpeg' # aerial view
url4 <- 'https://wmts.geo.admin.ch/1.0.0/ch.swisstopo.swisstlm3d-karte-grau.3d/default/current/3857/{z}/{x}/{y}.jpeg' # topographic greyscale map
# Create leaflet map using centroids #####################################################################
addLegendCustom <- function(map, color, labels, sizes, opacity = 1, stroke, title = "Observations per grid", position = "bottomright"){ # setup customised legend to plot circles (instead of squares)
colorAdditions <- paste0(color, "; border-radius: 50%; width:", sizes, "px; height:", sizes, "px")
labelAdditions <- paste0("<div style='display: inline-block;height: ",
sizes, "px;margin-top: 4px;line-height: ", sizes, "px;'>",
labels, "</div>")
return(addLegend(map,
colors = colorAdditions,
labels = labelAdditions,
opacity = opacity,
title = title,
position = position))
}
m <- leaflet(centroidsZZT) %>%
addTiles(urlTemplate = url1, group ="Swisstopo colour") %>%
addTiles(urlTemplate = url2, group ="Swisstopo greyscale") %>%
addTiles(urlTemplate = url3, group ="Aerial view") %>%
addTiles(urlTemplate = url4, group ="Topographic view") %>%
addCircleMarkers(~ZZT$decimalLongitude, ~ZZT$decimalLatitude, fillOpacity = 1, col = c("red"), weight = 2, radius=5, group = "ZZT - precise") %>%
addCircleMarkers(~centroidsZZT$x, ~centroidsZZT$y, fillOpacity = 0.5, col = c("red"), weight = 2, radius=5, group = "ZZT - presence in grid") %>%
addCircleMarkers(~centroidsZZT$x, ~centroidsZZT$y, fillOpacity = 0.5, col = c("red"), weight = 2, radius=levels(cut(rZZT@data@values, c(1,5,10,20,100,1000), labels=c(1,3,5,10,20))), group = "ZZT - observations per grid") %>% #plot data points proportionate to number of counts per grid
addCircleMarkers(~centroidsINFOFLORA$x, ~centroidsINFOFLORA$y, fillOpacity = 0.5, col = c("blue"), weight = 2, radius=5, group = "InfoFlora - presence in grid") %>%
addCircleMarkers(~centroidsINFOFLORA$x, ~centroidsINFOFLORA$y, fillOpacity = 0.5, col = c("blue"), weight = 2, radius=levels(cut(rINFOFLORA@data@values, c(1,5,10,20,100,1000), labels=c(1,3,5,10,20))), group = "InfoFlora - observations per grid") %>%#plot data points proportionate to number of counts per grid
addLayersControl(baseGroups = c("Swisstopo colour", "Swisstopo greyscale", "Aerial view", "Topographic view"), overlayGroups = c("ZZT - precise", "ZZT - presence in grid", "ZZT - observations per grid", "InfoFlora - presence in grid", "InfoFlora - observations per grid"), options = layersControlOptions(collapsed = FALSE)) %>%
addLegend(colors = c("red","blue"), position = "bottomright", labels = c("ZZT", "InfoFlora"), opacity = 1, title = c("Dataset")) %>%
addLegendCustom(color = "grey", labels = c("1-5","6-10","11-20","21-100","101-1000"), sizes = c(3,5,10,20,40), stroke = FALSE)
m
# Save the leaflet maps as HTML objects ##################################################################
saveWidget(m, file="historicalMAP_example_Adonis_aestivalis.html")
Here's what you get:
a) Mapping of (precise) herbarium locations
b) Mapping of grids where the species is/was present (red, herbarium data; blue, InfoFlora data)
c) Mapping of grids where the species is/was present (red, herbarium data; blue, InfoFlora data); the sizes of the circles are proportionate to the number of observations recorded per grid (see legend at the bottom right)
To be implemented or solved
- The InfoFlora database entails observations dating back to the 1970s and the United Herbaria Zurich Z+ZT also possess vouchers from this millennium. Accordingly, one should implement a time slider, to facilitate temporal comparisons and accommodate data from other databases (e.g. iNaturalist).
- To better assess the reasons for putative distributional changes through time (e.g. habitat loss, urbanisation), one should integrate the historical Dufour and Siegfried swisstopo maps. Further maps (e.g. land use, vegetation type, road network) are, of course, welcome! Having the opportunity to visualise two maps (from two different time periods) side-by-side might also be useful, but maybe it would be more elegant to establish a hypothetical distribution based upon all the observations and let the internet users choose which data they want to plot against it (e.g. only data from a given dataset or a given time period).
- In order to identify putative wrong determinations, one should be able to access any images (if available) pertaining to the mapped datapoints (see examples from the United Herbaria Zurich Z+ZT or iNaturalist). Generally, implementing dynamic popups with important information pertaining to the datapoints would be a nice feature!
- Logically, the code should be setup in such a way that data are being automatically retrieved from GBIF, so that one always constructs the most current map.
- I herewith compared data of only one species (Adonis aestivalis L.), but ideally internet users should be able to customise their map based on following criteria: plant species, administrative areas (cantons), data sources (national database, herbaria, citizen science platforms), etc.
- Herbaria should be able to integrate the code into their websites.
- Export functions (with copyrights) are needed.